CS 660: Combinatorial Algorithms
Dynamic Lists
[To Lecture Notes Index]
San Diego State University -- This page last updated Sept. 5, 1995

Contents of Dynamic Lists Lecture
- References
- Searching
- Self-Organizing Linear Search
- General Information and Restrictions
- Zipf's Law
- Lotka's Law
- 80% - 20% Rule
- Convergence to Steady State
- Known Algorithms and Analysis
Hester, James and Hirschberg, Daniel, "Self-Organizing Linear Search",
Computing Surveys, 17(3):295-311, September 1985.
Search for x in a list of n data items

Standard Solution
* Sort the list of data items (or create a binary search tree)
- Cost for general list is Theta(nlg(n))
* Now search for x
- Average and worst case cost is Theta(lg(n))
Hidden Assumptions
* We will perform more than one search
- Number of searches should be Omega(lg(n))
* All items in the list will be searched for with nearly the same frequency
Contrived Example
Assume we have a list of n items:

The a's are ordered by frequency of access
Probability of accessing item
:
P(
)
=
Probability of looking for an item not in the list is

Average cost
-
-
- U = set of all possible events
- P(e) = probability of event e
- C(e) = cost of event e
We have:
-
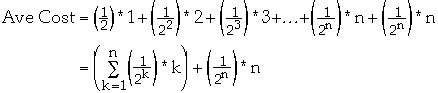
What is
?
We have:
-
-
-
So
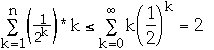
Thus Ave Cost =
Why linear search?
* Simple to code
location = -1
for (K = 0; K < n; K++)
if ( data[K].key == X) {
location = K;
break;
}
* Requires minimal space
Organizing the list
Assume we have a list of n items:

Probability of accessing item
* Optimal Static Ordering
* Move-to-front
* Transpose
Optimal Static Ordering
Assume P(ak) is known for all k in advance
Order items in decreasing probability
Example: 2.0 average comparisons
- Let P(a) = .2
- P(b) = .4 P(c) = .3 P(d) = .1
-
- Optimal static ordering
- b, c, a, d
Move-to-front
Start with any initial ordering
When item is accessed move it to the front of the list
Example: 2.2 average comparisons
a, b, c, d start order
b, a, c, d accessed b 2 comparisons
b, a, c, d accessed b 1
a, b, c, d accessed a 2
c, a, b, d accessed c 3
a, c, b, d accessed a 2
c, a, b, d accessed c 2
c, a, b, d accessed c 1
d, c, a, b accessed d 4
b, d, c, a accessed b 4
b, d, c, a accessed b 1
Transpose
Start with any initial ordering
When item is accessed move it forward one location
Example: 2.3 average comparisons
a, b, c, d start order
b, a, c, d accessed b 2 comparisons
b, a, c, d accessed b 1
a, b, c, d accessed a 2
a, c, b, d accessed c 3
a, c, b, d accessed a 1
c, a, b, d accessed c 2
c, a, b, d accessed c 1
c, a, d, b accessed d 4
c, a, b, d accessed b 4
c, b, a, d accessed b 3
Permutation algorithms
- Algorithm used to rearrange list after accessing a record
Restrictions
- Only consider permutation algorithms that move accessed item forward in the
list
-
- Will not search for items not in the list
-
- All items will be searched at least once
-
- Time required by any execution of the permutation algorithm is never more
than a constant times the time required for the search immediately before that
execution.
-
- Example
- Given the list
-
- Accessing second item requires two comparisons so permutation algorithm
can take c*2 time units
-
- Accessing the last item requires two comparisons so permutation algorithm
can take c*n time units
Measures of Performance
the search sequence
[k]
the item to be searched for on the k'th access
(
,
k) be the state of the list after the first k accesses from [[rho]]
(
,
k)r location in the list of item r after the first k accesses from [[rho]]
=
(
,
0) the initial configuration of the list
Cost of a permutation
for a given l and r is the average cost per access in terms of the number of
probes required to find the accessed record and the work required to permute
the records afterwards
Asymptotic Cost
- Average cost over all
and
for a given
-
- Usually restrict
to make analysis possible
Zipf noticed that in English the frequency of word usage follows:
-

where fi denotes the frequency of the ith most frequent word
Zipfian Probability Distribution:
Assume we have a list of n items:

The a's are ordered by frequency of access
Probability of accessing item
is
and

Then

But
so we have
-
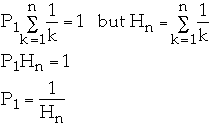
Zipfian Probability Distribution
Let
for k = 1, 2, ..., n
where

Pk
n = 2
k 1 2
Pk 0.6667 0.3333
n = 3
k 1 2 3
Pk 0.5455 0.2727 0.1818
n = 4
k 1 2 3 4
Pk 0.48 0.24 0.16 0.12
n = 5
k 1 2 3 4 5
Pk 0.438 0.219 0.146 0.109 0.0876
n = 6
k 1 2 3 4 5 6
Pk 0.408 0.204 0.136 0.102 0.0816 .0680
How to Implement Zipf's Distribution
Let
-
Method 1
If
<= rand() <
then return k
Method 2
The number of papers in a given journal written by the same author follows an
inverse square distribution.
Let n be the total number of authors who published at least one paper in a
given journal.
The probability that a randomly chosen author contributed exactly k papers is
given by:

n = 2
k 1 2
Pk 0.800 0.200
n =3
k 1 2 3
Pk 0.734 0.183 0.081
n =4
k 1 2 3 4
Pk 0.702 0.175 0.078 0.043
n =5
k 1 2 3 4 5
Pk 0.683 0.170 0.075 0.042 0.027
n =6
k 1 2 3 4 5 6
Pk 0.670 0.167 0.074 0.041 0.026 0.018
"80% of the transactions are on the most 20% of the records, and so on
recursively"
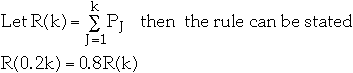
When n = 5*L we have:
-

n = 2
k 1 2
Pk 0.908 0.100
n = 3
k 1 2 3
Pk 0.858 0.100 0.063
n = 4
k 1 2 3 4
Pk 0.825 0.100 0.063 0.047
n = 5
k 1 2 3 4 5
Pk 0.800 0.100 0.063 0.047 0.038
80% - 20% Rule
Knuth claims we can approximate this by:

n = 2
k 1 2
Pk 0.644 0.355
n = 3
k 1 2 3
Pk 0.515 0.283 0.200
n = 4
k 1 2 3 4
Pk 0.446 0.245 0.173 0.135
n = 5
k 1 2 3 4 5
Pk 0.401 0.220 0.155 0.121 0.100
n = 6
k 1 2 3 4 5 6
Pk 0.369 0.203 0.143 0.111 0.092 0.078
Steady State
- Further permutations are not expected to change the expected search time
significantly
-
Locality
- Subsequences of [[rho]] may have relative frequencies of access that are
drastically different from the overall relative frequencies
-
-
-
Total Number of Comparisons in searchWorst Case
n Move-to-Front BST
7 112 170
15 360 490
31 1240 1290
63 4536 3210
Measures of Convergence
Relative Measurements
- Optimal Static Ordering - items are ordered by static probability of access
and are not moved
-
-
-
Total Number of Comparisons in searchWorst Case
n Move-to-Front OSO
7 112 210
15 360 1050
31 1240 4650
Move-to-front
-
- Assume Zipf distribution
-
-
-
-
-
-
Transpose
-
Count
- approaches optimal static ordering
Comparisons between Algorithms
No Optimal Memoryless algorithm
Asymptotic Cost
- Move-to-front asymptotic cost at most twice asymptotic cost of the optimal
static ordering
-
- Asymptotic cost of transpose is <= asymptotic cost of move-to-front
-
- Count is asymptotically equal to optimal static ordering
-
Worst Case
- Move-to-front and count at most twice the worst case of the optimal static
ordering
-
- Transpose can be far worse
-
- Moving a record any fraction of the distance to the front of the list will
be no more than a constant times the optimal off-line algorithm
-
- The constant is inversely proportional to the fraction of the total
distance moved